Vision & Offering
In this blog post we’re introducing Bitrock’s vision and offering in the Data, AI & ML Engineering area. We’ll provide an overview of the current data landscape, delimit the context where we focus and operate, and define our proposition.
This first part describes the technical and cultural landscape of the data and AI world, with an emphasis on the market and technology trends. The second part that defines our vision and technical offering is available here.
A Cambrian Explosion
The Data & AI landscape is rapidly evolving, with heavy investments in data infrastructure and an increasing recognition of the importance of data and AI in driving business growth.
Investment in managing data has been estimated to be worth over $70B [Expert Market Research 2022], accounting for over one-fifth of all enterprise infrastructure spent in 2021 according to (Gartner 2021).
This trend is tangible in the job market too: indeed, data scientists, data engineers, and machine learning engineers are listed in Linkedin’s fastest-growing roles globally (LinkedIn 2022).
And this trend doesn’t seem to slow down. According to (McKinsey 2022), by 2025 organizations will leverage on data for every decision, interaction, and process, shifting towards real-time processing to get faster and more powerful insights.
This growth is reflected also in the number of tools, applications, and companies in this area, and from what is generally called a “Cambrian explosion”, comparing this growth to the explosion of diverse life forms during the Cambrian period, when many new types of organisms appeared in a relatively short period of time. This is clearly depicted in the following figure, based on (Turk 2021).
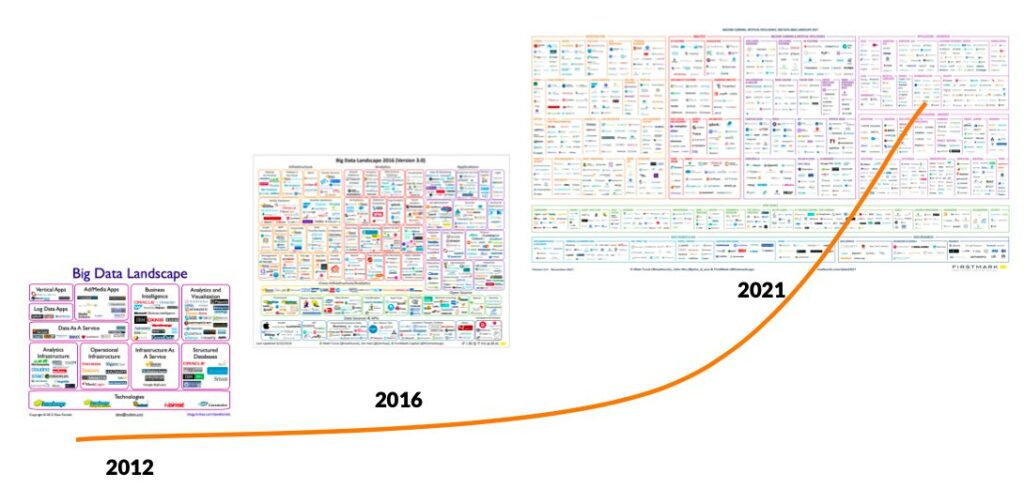
The Technological Scenario
Data architectures serve two main objectives, helping the business make better decisions exploiting and analyzing data – the so-called analytical plane – and provide intelligence to customer-facing applications – the so-called operational plane.
These two use-cases have led to two different architectures and ecosystems around them: analytical systems, based on data warehouses, and operational systems, based on data lakes.
The former, built upon data warehouses, have grown rapidly. They’re focused on Business Intelligence, business users and business analysts, typically familiar with SQL. Cloud warehouses, like Snowflake, are driving this growth; the shift from on-prem towards cloud is at this point relentless.
Operational systems have grown too. These are based on data lakes; their growth is driven by the emerging lakehouse pattern and the huge interest in AI/ML. They are specialized in dealing with unstructured and structured data, supporting BI use cases too.
Since a few years ago, a path towards a convergence of both technologies has emerged. Data lake houses added ACID transactions and data-warehousing capabilities to data lakes, while warehouses have become capable of handling unstructured data and AI/ML workloads. Anyway, the two ecosystems are still quite different, and may or may not converge in the future.
In the ingestion and transformation sides, there’s a clear architectural shift from ETL to ELT (that is, data is firstly ingested and then transformed). This trend, made possible by the separation between storage and computing brought by the cloud, is pushed by the rise of CDC technologies and the promise to offload the non-business details to external vendors.
In this context Fivetran/DBT shine in the analytical world (along with new players like airbyte/matillion), while Databricks/Spark, Confluent/Kafka and Astronomer/Airflow are the de-facto standards in the operational world.
It is also noteworthy that there has been an increase in the use of stream processing for real-time data analysis. For instance, the usage of stream processing products from companies such as Databricks and Confluent has gained momentum.
Artificial Intelligence (AI) topics are gaining momentum too, and Gartner, in its annual report on strategic technological trends (Gartner 2021), lists Decision Intelligence, AI Engineering, Generative AI as priorities to accelerate growth and innovation.
Decision Intelligence involves the use of machine learning, natural language processing, and decision modelling to extract insights and inform decision-making. According to the report, in the next two years, a third of large organisations will be using it as a competitive advantage.
AI Engineering focuses on the operationalization of AI models to integrate them with the software development lifecycle and make them robust, reliable. According to Gartner analysts, it will generate three times more value than most enterprises not using it.
Generative AI is one of the most exciting and powerful examples of AI. It learns the context from training data and uses it to generate brand-new, completely original, realistic artefacts and will be used for a multitude of applications. It will account for 10% of all data produced by 2025 according to Gartner.
Data-driven Culture and Democratization
Despite the clear importance of data, it’s a common experience that many data initiatives fail. Gartner has estimated that 85% of big data projects fail (O’Neill 2019) and that through 2022 only 20% of analytic insights will deliver business outcomes (White 2019).
What goes wrong? Rarely problems lie in the inadequacies of the technical solutions. Technical problems are probably the simplest. Indeed, since ten years ago, technologies have evolved tremendously fast and Big Data technologies have matured a lot. More often, problems are rather cultural.
It’s not a mystery that a data lake by itself does not provide any business value. Collecting, storing, and managing data is a cost. Data become (incredibly) valuable when they are used to produce knowledge, hints, actions. To make the magic happen, data should be accessible and available to everybody in the company. In other words, organizations should invest in a company-wide data-driven culture and aim at a true data democratization.
Data should be considered a strategic asset that is valued and leveraged throughout the organization. Managers, starting from the C-levels, should remove obstacles and create the conditions for people in need of data to access them, by removing obstacles, bottlenecks, and simplifying processes.
Creating a data culture and democratizing data allows organizations to fully leverage their data assets and make better use of data-driven insights. By empowering employees with data, organizations can improve decision-making, foster innovation, and drive business growth.
Last but not least, Big Data’s power does not erase the need for vision or human insight (Waller 2020). It is fundamental to have a data strategy in mind to define how the company needs to use data and the link to the business strategy. And, of course, a buy-in and commitment from all management levels, starting from the top.
The second part of this article can be found here.
References
- Expert Market Research. 2022. “Enterprise Data Management Market Size, Share, Price, Demand 2023-2028.” Expert Market Research. https://www.expertmarketresearch.com/reports/enterprise-data-management-market.
- Gartner. 2021. “Gartner Identifies the Top Strategic Technology Trends for 2022.” Gartner. https://www.gartner.com/en/newsroom/press-releases/2021-10-18-gartner-identifies-the-top-strategic-technology-trends-for-2022.
- Gartner. 2021. “Forecast: Enterprise Infrastructure Software, Worldwide, 2019-2025, 4Q21 Update.” Gartner. https://www.gartner.com/en/documents/4009669.
- LinkedIn. 2022. “The Fastest-Growing Jobs Around the World.” LinkedIn. https://www.linkedin.com/business/talent/blog/talent-strategy/fastest-growing-jobs-global.
- McKinsey. 2022. “The data-driven enterprise of 2025.” McKinsey. https://www.mckinsey.com/capabilities/quantumblack/our-insights/the-data-driven-enterprise-of-2025.
- O’Neill, Brian T. 2019. “Failure rates for analytics, AI, and big data projects = 85% – yikes!” Designing for Analytics. https://designingforanalytics.com/resources/failure-rates-for-analytics-bi-iot-and-big-data-projects-85-yikes/.
- Turk, Matt. 2021. “Red Hot: The 2021 Machine Learning, AI and Data (MAD) Landscape.” Matt Turck. https://mattturck.com/data2021/
- Waller, David. 2020. “10 Steps to Creating a Data-Driven Culture.” Harvard Business Review. https://hbr.org/2020/02/10-steps-to-creating-a-data-driven-culture.
Author: Antonio Barbuzzi, Head of Data, AI & ML Engineering @ Bitrock